SAE Latent Co-occurrence
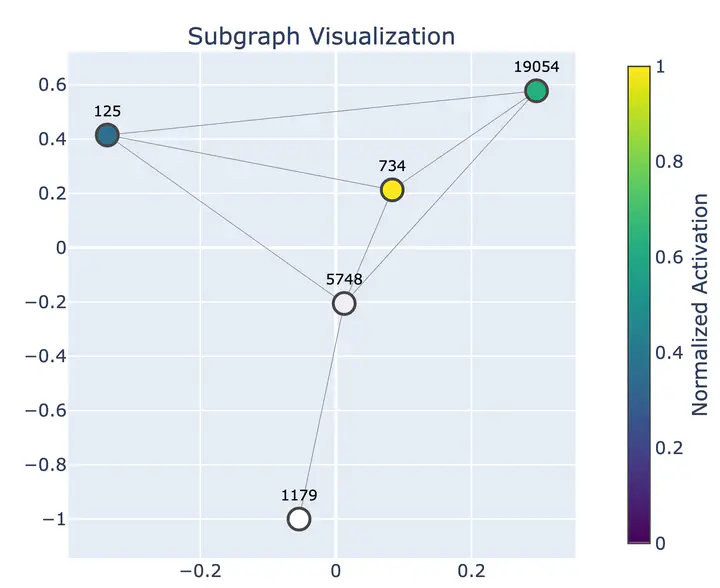
Sparse AutoEncoder (SAE) latents show promise as a method for extracting interpretable features from large language models (LM), but their overall utility as the foundation of mechanistic understanding of LM remains unclear. Ideal features would be linear and independent, but we show that there exist SAE latents in GPT2-Small display non-independent behaviour, especially in small SAEs. Rather, they co-occur in clusters that map out interpretable subspaces. These subspaces show latents acting compositionally, as well as being used to resolve ambiguity in language. However, SAE latents remain largely independently interpretable within these contexts despite this behaviour. Furthermore, these clusters decrease in both size and prevalence as SAE width increases, suggesting this is a phenomenon of small SAEs with coarse-grained features.
For more, see our post on LessWrong, my talk as part of the PIBBSS 2024 summer symposium or explore the SAE Latent Co-occurrence App.